Is there a way to determine what data is where on your network, and which documents contain which keywords? Even worse, what if an attacker could find the precise documents by doing an automated search?
Speaking to Infosecurity ahead of his talk at RSA Conference, Etienne Greeff, CTO of Secure Data, discussed the concept of using machine learning to scan a network to find key words, and how it can be done in reverse.
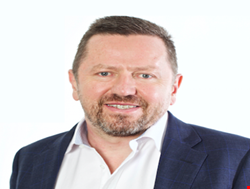
Explaining how the concept works, Greeff said it is based on what he called “topic modelling,” which is an unsupervised learning algorithm that allows you to look at a large set of text and figure out which words occur the most, and which words occur together the most.
“So what that allows you to do, is if I have a bunch of documents I can quickly and accurately discern what topics are being discussed in those documents,” he said. “Let’s say on a network I can figure out what's discussed on a network or on an endpoint, and determine the user to be a financial guy or an accountant, and with a high degree of accuracy, figure out what it is about.”
In order to turn this into a weapon, Greeff said you would create a watering hole attack and entice the target to go to that website, and download a topic modelling payload. “On my command and control I can determine the words I care about, and only extract documents that only talk about passwords, patents, copyright and filing documents. So I don’t need to know anything about the user or the documents, just get what I care about in an automated fashion.”
He explained that the watering hole will deploy the payload onto the network, and categorize the documents that relate to those key words. This would allow an attacker to engineer the watering hole and extract documents.
“So you can quite accurately extract documents, without any knowledge of the network.”
Greeff described it as a form of “reverse DLP” as you use machine learning to sift quickly through documents. Infosecurity asked if this could enable some sort of Big Data analysis, which Greeff agreed with, saying that topic analysis is commonly used by libraries or news aggregators, but this version of weaponizing it allows an attacker to use it for nefarious means.
Greeff claimed that machine learning is more suited to the attack of a network than for defending an organization “but there are not that many offensive uses of machine learning.” While there are uses of image manipulation to alter machine learning, and there are examples of creating text to confuse AI, many are very academic and do not scale to the business.
“So we decided to create a machine learning tool that you could use as a hacking tool in scalable, repeatable and automated fashion, and I feel we succeeded as it is a totally different form of attack as it is a new way to think about how to attack a network.”
So what about defending against this? Greeff was not confident that it was easy to defend against, saying that every DLP vendor uses “regular expressions” or hashes to determine what is leaving the network. “Everybody uses very elementary ways of determining what is leaving the network, but if you do topic modelling you can quite accurately discern what topic is in what document.”
He said that you can do topic modelling “on the fly” to understand where sensitive documents are on the network, and figure out what topics are leaving your network “without having to rely on pattern matching, certain words or snippets of stuff.”
The use of topic modelling is something every DLP vendor should be doing, he claimed, adding that it gives you a much better way of detecting what is leaving and more granular visibility.
So why do this now? Greeff said that it came from his own interest, and he believed that it is the first scalable attack using machine learning to get to data in a very efficient way in a victim’s network.
Asked if this was a way to build a better DLP, Greeff said that this could be the future of DLP as if it used the same techniques to attack networks, “DLP would be much more efficient, and you would not have to configure as much as you do with current DLP.”
He concluded by saying that the way to think about this is as a “data-driven attack” and not a technical attack, as an attacker can determine the sort of data they are interested in, and using machine learning, they can extract that data quite efficiently.
“In the past it is a very manual job, as the attacker has to get on the network and get a bunch of documents and manually review each of the documents to see which ones they care about, and that just doesn’t scale. With this, it is scalable.”