In 1950, pioneering computer scientist Alan Turing developed the ‘Turing Test’ with the aim of gauging a machine’s ability to exhibit intelligent behavior equivalent to, or indistinguishable from, that of a human. In essence, Turing wanted to discover whether machines had the ability to ‘think’ for themselves. Almost 10 years later, American computer gaming and artificial intelligence innovator Arthur Lee Samuel coined the term ‘machine learning’, which he called a “Field of study that gives computers the ability to learn without being explicitly programmed.” Fast-forward to the 1990s, work on machine learning shifted from a knowledge-driven approach to a data-driven one, with scientists building computer programs designed to draw conclusions by analyzing large amounts of information. The point being, the concept of cognitive computers is not new.
However, we find ourselves in an age where recent technological advances and the explosion of available data have opened the doors to a wealth of new possibilities in what machine learning has to offer, inflating expectations as a result. From creating systems to aid doctors in giving more effective or accurate diagnoses, developing autonomous and more efficient transport, driving innovations in the field of science, to voice and face recognition systems, this branch of artificial intelligence (AI) impacts our daily lives more now than ever before.
“Increasingly affordable processing power coupled with inexpensive storage is how these algorithms have become more relevant to looking at patterns in everyday life,” Davi Ottenheimer, president of flyingpenguin, tells Infosecurity. “They are used to build models that ‘expose’ insights and connections in all the data being collected by the sensors and systems you find around you.”
It is, therefore, unsurprising that the notion of applying machine-based learning methods to information security has also gathered significant pace over the last few years, with businesses, vendors and users alike turning an eye to leveraging these evolving algorithms for the benefit of protecting data.
“Machine learning can be a powerful tool for cybersecurity,” says John Bruce, CEO of Resilient. “We face increasingly complex cyber-attacks, complicated business and technology environments and a widening skills gap.
“Cognitive solutions that use machine learning and are integrated into an orchestrated security response function have enormous potential.”
So how is that potential being implemented in today’s security landscape, and where is it heading?
A Progressive Approach
Speaking to Infosecurity, Microsoft UK’s national security officer Stuart Aston explains that the cyber-threat environment has become so advanced that disruptive technologies such as machine learning need to be incorporated within a company’s cybersecurity strategy.
“It’s essential for organizations to be brave and implement a progressive security model to keep up with the latest threats as they emerge,” he argues. “Machine learning has a key role to play in advancing the way organizations protect themselves and move to a progressive approach.”
This involves taking advantage of data relating to attacks that is already flowing through an organization’s system, he adds, by using analytics engines to drive threat intelligence and harness machine learning to create a system that learns from itself and evolves in real-time.
“At Microsoft,” Aston continues, “we’ve woven intelligence throughout our security platform to bolster the protection of identities, apps, data and devices; empowering our customers to eradicate potential threats with comprehensive and rapid insight” by detecting suspicious activity and identifying attempts to access sensitive data.
The software then goes one step further, notifying designated security administrators and providing recommendations on how to mitigate certain threats.
“This constant evolution will help organizations to stay ahead of attackers and enable systems to learn to recognize threat patterns faster to ensure a quick response.”
“Cognitive solutions that use machine learning and are integrated into an orchestrated security response function have enormous potential”
He Who Learns Quickest
This then brings us onto the element of speed. Organizations today are faced with handling, aggregating and synthesizing unpredicted amounts of data not only for business success but also in a secure and safe manner, which means needing the ability to rapidly pick up and act upon abnormalities and threats. Yet, the reality is, it’s practically impossible for the vast majority of businesses to even make sense of so much data on-premises and turn it into security, let alone doing so quickly.
Nonetheless, in a business world where competition is rife, the notion of ‘he who learns quickest wins’ carries a significant amount of weight, and it’s that thought process which is driving much of the uptake of machine-based security approaches in more and more companies.
Bruce describes how machine learning can be used to save time in a period where businesses are taking longer to respond to attack (recent global research from the Ponemon Institute found that attack response times had increased in 41% of the surveyed organizations) and dealing with increasingly demanding workloads with limited manpower and resources.
“Low-level response actions that frequently occur can be automated, such as SIEM queries, threat intelligence lookups or IT ticket creation. Doing so ensures accuracy, offloads work when possible and increases response speed”, he says.
Likewise, another effect is identifying false positives, Bruce continues: “According to the 2015 IBM Cyber Security Intelligence Index, organizations spend an average of 21,000 hours each year identifying false positives.” Machine learning platforms, such as IBM Watson, help identify these false positives more efficiently.
“If you think about how much time gets put into tracking down a security alert, it can be hours and hours and sometime days, meanwhile the attack is going on in real time,” adds Mike Banic, vice-president of marketing at Vectra. “With machine learning, we can train it to look for very specific features that help the security analysts know whether an attacker has his fingers on the keyboard controlling the attack with a remote access trojan, or if the attacker has landed on a host and is performing reconnaissance, or if the attacker has stolen someone’s credentials to get closer to sensitive data.”
All of these things would only normally be discovered by spending hours/days of man hours based on seeing little signals, Banic says, whereas with machine learning, it is all done in real time. The machine learning does the things that are very mundane and time-consuming, empowering the security analyst to actually get ahead of the attack.
"Machine learning has a key role to play in advancing the way organizations protect themselves and move to a progressive approach”
A Question of Value
What’s clear is that companies should (and in many cases need to) be open to exploring the possibility of implementing machine learning and all the benefits it can bring to an organization’s security strategies. Speed, efficiency, threat disruption and detailed detection – the potential pluses are plentiful and there’s certainly a lot to whet the appetite of those looking to use machine learning to bolster their security.
However, to do so effectively, what’s imperative is also maintaining a focus on questioning its value in relation to a variety of different factors.
“First of all, companies need to understand why and what they are implementing machine learning for,” Ilia Kolochenko, CEO of web security company High-Tech Bridge, tells Infosecurity. “If a company does not have a clear vision of its direct benefits, machine learning is not only useless but can also be harmful. Also, machine learning can be quite expensive due to required processing and storage capacities. Therefore, if you efficiently and effectively solve your current problems without machine learning – continue to do so.”
What’s more, as Oliver Tavakoli, CTO of Vectra Networks, discussed at this year’s Infosecurity Europe in London, it’s also vitally important to fully understand the value of your data in terms of its origin and legitimacy. There is potential for it to be polluted by attackers who purposefully target security systems based on machine learning. Their intention is to disrupt the algorithm’s ability to draw correct and accurate conclusions, resulting in flawed results.
“With machine learning, what you are doing is having the data train the program,” he said at the event. “So the data can train the algorithm but it can also fool the algorithm. You have to be really careful about where you get your data from.”
This means companies looking to purchase ready-made machine-based learning platforms need to be asking what data is being used as input, where it’s from and be assured as to the data’s purity. Those opting to build their own, however, need confidence in their data science teams; ensuring the data acquisition process has integrity and knowing whether data includes the right features to detect appropriate use cases.
Further and perhaps most poignantly, Aston adds, machines and humans must work together to be successful, not against each other. He draws attention to the ongoing value of the human and our relationships with these autonomous algorithms.
“Human experts have an increasingly important role to play in this process of protecting customers, in tandem with machine learning,” he says.
“We humans have creativity, empathy, emotion, physicality and insight that can then be mixed with powerful AI computation – affording the ability to contextualize large amounts of data. This again highlights the human element and technical expertise required to get the most out of data, emphasizing how critical the role of highly trained professionals in this area will be in years to come.”
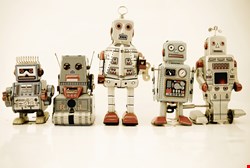
Rage Against the Machine?
It is perhaps this particular human-related element that Aston describes which steers thoughts towards arguably the most important and troubling issue to have raised its head regarding machine learning technology: ethical bias and the inability of computers, regardless of their processing power, to make ethical decisions akin to that of the human mind.
Issues such as cost, business value and data authenticity are all important things to consider, but as Ottenheimer explains, this is one of the biggest problems currently surrounding machine learning.
“Machine learning is being used in ways that fail to consider implicit bias, leading to unethical results and confusion about accountability for harm”, he warns.
Ottenheimer points to real-life instances where autonomous decisions have been made which, he argues, raise ethical concerns. For example, online searches for ‘professional hairstyle’ returning only images of white people, ‘false’ criminal labeling based on racial stereotyping and fatal motor vehicle collisions caused by false expectations about cruise control and lane assist capabilities.
“The dangers of racism [for example] are self-explanatory. Less obvious is that machines speed up the mistakes humans make and are less able to correct their way out of errors.
“You could see, for example, a machine making 100-times the errors and continuing to ‘learn’ on destructive/harmful ways instead of realizing it is doing bad things.”
If anything, this truly reinforces just how important human input is going to be as machine learning technologies continue to evolve and become more powerful. Whilst Tavakoli argues that “every technology can raise ethical questions”, it’s vital we understand that such flaws can find their way, even inadvertently, into this technology and that we, as security professionals, ensure they are empathetically and reflectively managed, rectified and unable to cause unwanted harm as we bring them into the cybersecurity landscape.
“Like any automation tool that can be used for good/bad purposes, the trick with security is to set learning on the right path and manage the output,” Ottenheimer adds. “A fair way of looking at it is to consider machine learning like a child and the person operating it like a parent. We need to think more carefully about how we teach anything – machine or human – to avoid and remove bias ahead of decisions instead of waiting for disaster and playing dumb.”
A Sidekick, Not a Super Hero
It seems like Bill Gates was onto something when he said “A breakthrough in machine learning would be worth ten Microsofts”, and whilst it remains to be seen when (or if) the phenomena of machine learning will reach its true and full potential (Tavakoli argues that “we’re no further than 25% of the way there – and that’s being shockingly optimistic”) there are already some impressive security benefits to gain from using it.
“Over the coming years, this technology is set to become even more intelligent, develop further and revitalize the security industry with new techniques and possibilities,” Aston says. “The benefits of using this type of technology will become apparent to those not currently utilizing.”
However, machine learning should not be viewed as a security solution; it’s very much a clever type of support – more of a sidekick, and not a super hero. For some time we have pondered whether the arrival of machines that can increasingly carry out tasks conventionally done by people would eventually make humans less relevant, and whilst in some sectors that may prove to be the case, information security appears to be a ‘bottomless pit’ of change and evolution in which machine learning and AI will actually empower the security professional, instead of replacing them.
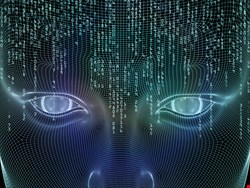
Anything You Can Do
As we explore how machine learning is being used for security in the enterprise, we cannot ignore the ways in which malicious forces are also adopting the technology
for themselves.
“Cyber-criminals mainly use machine learning for various classification tasks aimed to improve victim selection and targeting,” says Ilia Kolochenko. “They also use some elements of machine learning to identify valuable data in large amounts of stolen documents.”
What’s more, criminal enterprises and nation states that wish to attack you have a very high incentive to do so and, as Oliver Tavakoli explains, have actually proven to be more likely to adopt new forms of technology to do so than some organizations.
“They are quick to adopt tech and there is always a new criminal element coming along. You’re going to have to keep ‘arming up’ because you’re dealing with an adversary that’s using many of the same tools. The notion of not using machine learning is seeding that tool to your adversary – which is not really
an option.”