The emergence of powerful language models and their companion chatbot tools like OpenAI’s ChatGPT and Anthropic’s Claude has revolutionized human-computer interaction, but their rapid development has also raised critical concerns about data privacy.
As these models become increasingly sophisticated, the potential for misuse of personal information grows exponentially.
Recently, Manchester-based law firm Barings Law has rallied 15,000 people to sue Google and Microsoft over numerous alleged violations of data misuse relating to their AI models.
The law firm claimed to have found evidence that significant amounts of the data the two tech giants collected were being used in training and developing AI models without proper authorization or consent from users.
This episode is the latest in a flurry of conflicts over data privacy violations by GenAI providers over the past two years.
Matt Cooke, Cybersecurity Strategist for EMEA at Proofpoint, said that AI, and particularly GenAI, has introduced significant data loss risks.
“Inputting confidential information or Personally Identifiable Information (PII) into these models is like handing attackers a loaded weapon, and organizations are understandably worried,” he commented.
Chinese Technology Intensifies Concerns Over Data Protection
With the recent arrival of DeepSeek, a cutting-edge AI model developed in China, these privacy concerns have intensified significantly.
Noyb, the Austria-based European Center for Digital Rights, recently filed complaints against six Chinese companies (AliExpress, Shein, Temu, TikTok, WeChat and Xiaomi) over alleged violations of the EU’s General Data Protection Regulation (GDPR).
The non-profit noted, “Given that China is an authoritarian surveillance state, it is crystal clear that it doesn’t offer the same level of data protection as the EU.”
Noyb, which has criticized US GenAI providers regarding alleged data violations in the past, will likely start investigating DeepSeek over data privacy concerns.
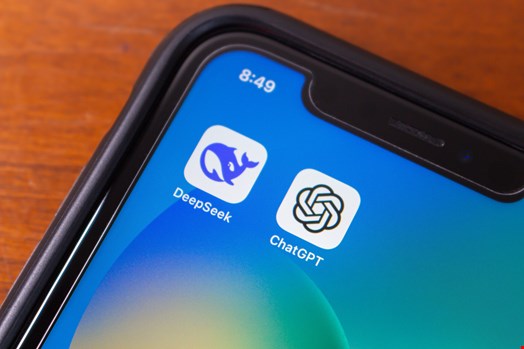
Data Exposure Concerns Slow Corporate GenAI Adoption
Meanwhile, the interest in adopting AI and GenAI tools in the workspace is progressing rapidly.
According to a CrowdStrike survey published in December 2024, 64% of IT and security professionals are either researching GenAI tools or have already purchased one.
Additionally, 70% of respondents said they intend to make a GenAI purchase within the next 12 months.
Adoption, on the other hand, is growing much more slowly, with only 6% of respondents in the CrowdStrike survey having actually implemented a GenAI tool and just 18% actively testing one.
This caution can be primarily attributed to data privacy concerns, with sensitive data exposure to underlying large language models (LLMs) being the most cited reason for delaying or limiting their adoption of GenAI tools.
According to Proofpoint’s Cooke, this survey resonates with his company’s 2024 Voice of the CISO report, which “revealed that 44% of UK CISOs identify GenAI tools as a top organizational risk, underscoring the need for robust data protection strategies.”
Infosecurity has selected five key strategies IT and security leaders can adopt to mitigate data exposure from GenAI tools and ensure the protection of sensitive and personal information.
Top Five Strategies to Mitigate Data Privacy Risks from GenAI
Data Minimization and Anonymization: Use Only What's Necessary
Cooke emphasized the human element in data loss, highlighting that "data doesn't lose itself; data loss originates with people." This underlines the importance of minimizing the data GenAI models require.
Two primary steps It and security leaders can take include:
- Anonymizing data whenever possible: Remove personally identifiable information (PII) like names, addresses, and phone numbers. Techniques like differential privacy can add noise to data while preserving patterns
- Generating artificial data that mimics real-world scenarios without containing actual PII. However, some experts argue that relying too much on this technique can create the risk of model collapse, a phenomenon where machine learning (ML) models gradually degrade due to errors coming from uncurated training on the outputs of another model
Fight Bias with Diverse Datasets and Active Detection
Biases in GenAI models can have a disproportionate impact on certain groups. Here's how to combat them:
- Train on diverse datasets: Ensure your training data represents a variety of demographics and backgrounds to minimize biases related to gender, race, and other factors
- Conduct regular bias audits and mitigation techniques: Sunil Agrawal, CISO at Glean, highlighted the need for "real-time detection and correction mechanisms" to address bias as it arises. Regularly audit your models and implement techniques like adversarial debiasing to identify and mitigate bias
Transparency and Accountability: Building Trust with Users
Transparency is key to building trust with users about how their data is used in GenAI systems.
To ensure that their workforce is using the corporate GenAI tools in a vetted manner, IT and security leaders should implement the following measures:
- Publish clear and accessible privacy policies: Disclose how user data is collected, used and protected by their GenAI systems and tools
- Protect data subject rights: Empower users with the right to access, correct and delete their data
- Enable internal accountability mechanisms: Designate individuals or teams responsible for data privacy compliance within their organization
Robust Data Security: Building Fortresses Around Your Data
Strong security measures are essential to protect sensitive data used in GenAI development and deployment. Here are two examples of robust security measures that could be implemented to ensure secure GenAI adoption in the workspace:
- Secure data storage and access: Utilize robust encryption, access controls and multifactor authentication (MFA) to safeguard data at rest and in transit
- Regular security audits and penetration testing: Identify and address potential vulnerabilities in the data security infrastructure through regular audits and testing
Stay Informed and Adapt: Keeping Pace with the Evolving Landscape
The world of data privacy regulations and best practices is constantly evolving. Here's how to stay ahead of the curve:
- Stay informed about new privacy regulations: Keep up with changes in regulations like GDPR, the California Privacy Rights Act (CPRA) and emerging AI-specific regulations such as the EU AI Act
- Regularly review and update privacy policies and procedures: Adapt your practices to reflect evolving technologies and regulatory requirements.
Conclusion
By implementing these strategies, organizations can harness the power of GenAI while minimizing data privacy risks.
IT and security professionals should remember that data privacy needs to be embedded in the development and deployment of GenAI systems from the beginning.