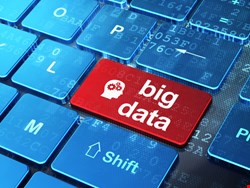
As security attacks become both more persistent and complex, the rules-based approach to network and data protection – where security information and event management (SIEM) systems are designed to track and mitigate ‘known threats’ – is becoming increasingly ineffective.
What happens when a new and previously unidentified piece of malware comes calling? Once it’s inside the network, an attack can ensure that evidence of its presence is hidden in the massive amounts of ‘normal’ data that enterprise systems generate – and trying to locate it is extremely difficult.
That’s why the new frontier of enterprise security is statistical analysis and pattern recognition in Big Data – specifically, machine data. This is the unstructured maze of system logs and transactions generated whenever humans interact with machines – such as web applications, email, network devices, external websites, mobile devices, sensors – or the large amounts of data generated by machines communicating with each other.
In this new world of security, CSOs/CISOs and IT teams have to unlearn their over-reliance on traditional data protection technologies such as anti-virus software, firewalls, and SIEM systems. The non-stop barrage of attacks that the enterprise faces has turned security into a reactive, administrative role, where team members are just responding to system alerts rather than applying their knowledge and thinking more laterally about threats.
Security should be an exciting industry to work in, but too often, both seasoned professionals and new entrants aren’t challenged intellectually – they just do what they’re told to do by the tools they use.
To address this, security professionals need to have much greater oversight of everything that’s happening inside the enterprise. They also need the ability to quickly analyze and sift through the machine data generated by interactions with IT systems in order to identify unusual patterns and abnormal behaviors that could indicate an attack is taking place.
Although Big Data analysis technologies exist that can help identify possible anomalies, it still requires human insight and intelligence to interpret what they might mean.
For example, the presence of URL strings that are four to five times longer than normal could indicate the possible presence of command-and-control instructions attempting to launch a web protocol attack. Another ‘tell’ could be a network access password being entered 10 times faster than it’s possible for a human to type. Or an excessive amount of outbound DNS traffic or DNS requests could indicate that an employee’s machine has become part of a botnet.
Interrogating machine data is also an excellent way of spotting when a security threat is being created internally – not by a clever piece of malware, but by a malicious insider who may feel entitled to intellectual property and wants to take it with them to their new job. Questions you could answer by analyzing data are: Why is a user repeatedly trying to access a file they don’t have permission to view; why is there a significant change in the mix of categories of websites they surf to; or why has their ID card been used to enter the office when they’re meant to be on holiday in the Bahamas?
Over the last few years, Big Data security platforms have emerged as a new weapon for forward-thinking organizations. These platforms are helping to level the playing field and make it possible to detect advanced threats early by being able to scale up to 100 terabytes or more per day and ingest all types of machine data, without a SQL datastore or fixed schema. These platforms also leverage distributed search for fast, real-time alerting and use statistics to spot anomalies and deviations, as well as scale horizontally by adding more indexers or nodes and installing on commodity hardware.
In addition, these platforms can not only help spot advanced threats, they can also be used for forensics, incident investigations, fraud detection and other non-traditional IT use cases. For example, data from industrial control systems, HVAC systems, GPS information or RFID data can be used to monitor the integrity of drug manufacturing or the movement of goods in a just-in-time manufacturing supply chain – risks beyond those traditionally monitored.
Achieving this level of operational intelligence not only opens up new possibilities for how companies defend themselves against myriad security threats they face, but also re-engages the interest and creativity of the IT teams entrusted with the task of overall risk mitigation.
The days of rules-based security engines are drawing to a close because they’re simply not built to handle the volume and sophistication of today’s attacks, and they can’t give businesses insight into risk. To truly understand the nature of the threats they face, organizations need to move beyond traditional approaches to security and delve deeper into the machine data they generate every second of every day.
Although there may not be a silver bullet for advanced threat detection, Big Data represents a compelling way to change the tide of online warfare against the cybercriminals.
Mark Seward, CISA, is currently senior director, Security and Compliance, at Splunk Inc. He has over 10 years of experience in the IT security management profession as a security practitioner and product manager with experience in log management and vulnerability management, including extensive security work for the US Treasury Department. Seward holds an MS in information technology and a Federal CIO certification from the University of Maryland.